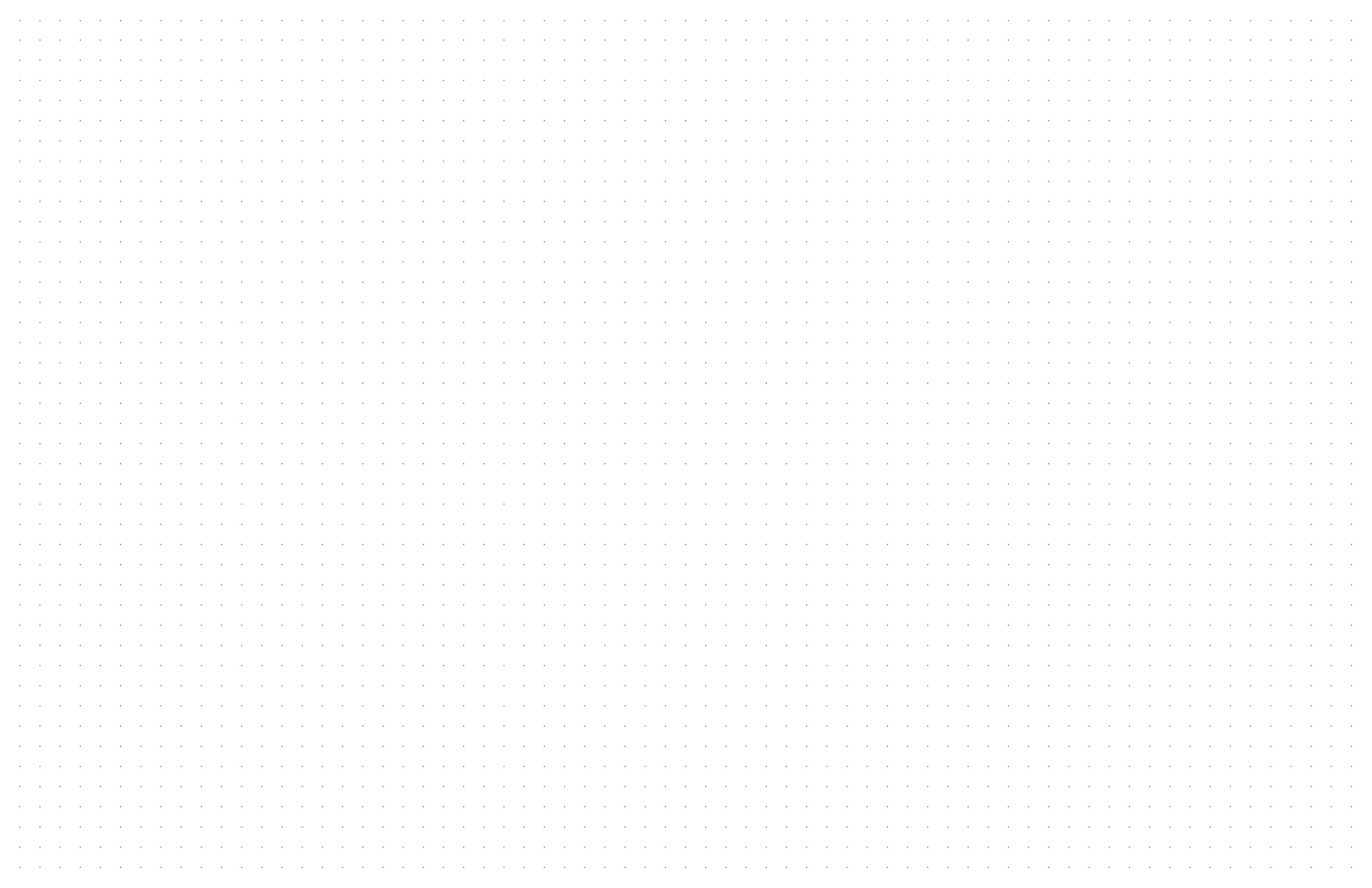
Evidence-based machine learning algorithms
PulseAI’s technology is built upon years of peer-reviewed scientific and clinical research in artificial intelligence, cardiology and digital signal processing.
Clinical Validation of a Chest-Worn Personal ECG Device for the Detection of Atrial Fibrillation
Circulation, Supplement 1, 2024
Cardiovascular Digital Health Journal, 2024
Precordial electrocardiographic recording and QT measurement from a novel wearable ring device
Cardiovascular Digital Health Journal 2024
Computing in Cardiology Conference, 2023
Comparison of reduced lead sets in detection of common ECG abnormalities
Journal of Electrocardiology, 2023
European Society of Cardiology Congress 2023
Novel AI algorithm improves the automated detection of Atrial Arrhythmias from the Apple Watch
Heart Rhythm 2023
Computing in Cardiology Conference, 2022
Automated Identification of Label Errors in Large Electrocardiogram Datasets
Computing in Cardiology Conference, 2022
Automated Measurement of the Heart-Rate Corrected QT-Interval using Deep Learning.
46th International Society of Computerized Electrocardiology Conference 2022
Device agnostic AI-based analysis of ambulatory ECG recordings
Journal of Electrocardiology, 2022
A two-staged classifier to reduce false positives: On device detection of atrial fibrillation using phase-based distribution of poincaré plots and deep learning.
Journal of Electrocardiology, 2022